Computers Can Sense Sarcasm? Yeah, Right
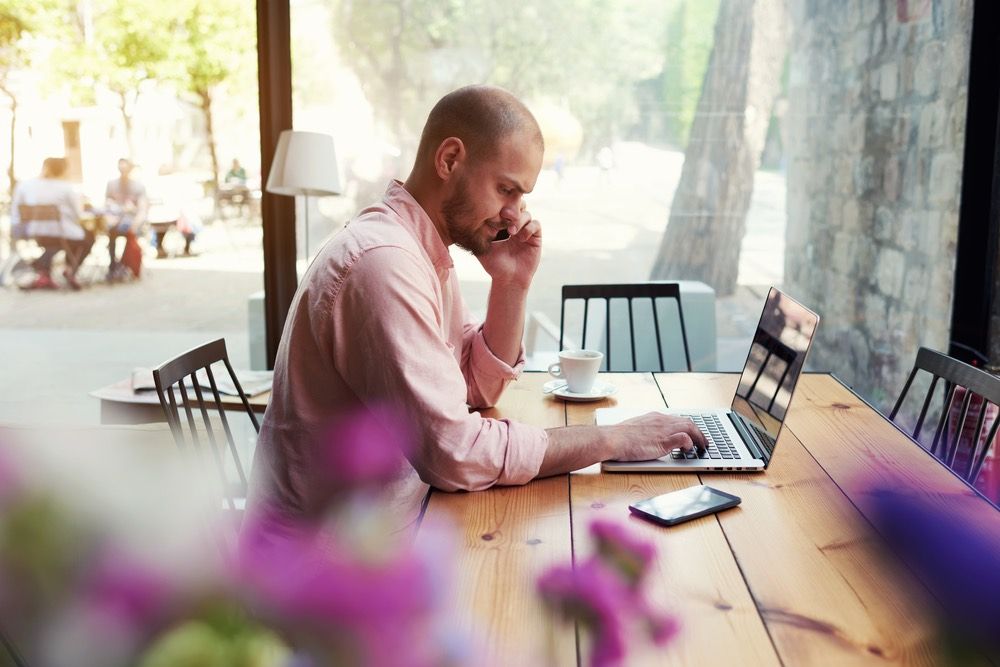
Humans pick up on sarcasm instinctively and usually do not need help figuring out if, say, a social media post has a mocking tone. Machines have a much tougher time with this because they are typically programmed to read text and assess images based strictly on what they see. So what's the big deal? Nothing, unless computer scientists could help machines better understand wordplay used in social media and on the internet. And it looks like they may be on the verge of doing just that.
Just what you needed—a sarcasm-detection engine that helps marketers tell whether you were praising or mocking their product, and adjust their messages to sell you more stuff. Yet promoters say savvier computers could also help law enforcement agencies distinguish legitimate threats from those that exaggerate or poke fun at serious topics, especially in Twitter, Instagram and Tumblr posts that use images. It might even help automated customer service systems figure out that you're upset, and route you to a real person or allow politicians to sense whether their messages are resonating with voters.
Rossano Schifanella, an assistant professor in computer science at the University of Turin, and a group of colleagues from internet company Yahoo! are trying to teach machines that humans do not always mean exactly what they say. What is new about their research, released earlier this month on the science publishing site ArXiv, is that they examined images as well as text in looking for clues to understand meaning. "What we observed is that if you just look at text, it isn't enough," Schifanella says. "The images provide crucial context."
Convinced that sarcasm really is a big deal, Schifanella points out that a company or institution could use automated mockery detection to better gauge public sentiment about its products or image. For example, Republican presidential candidate Donald Trump's staff could have saved the campaign a lot of grief if they had tested the Trump–Pence logo on social media before officially releasing it. The Twitterverse had a field day with the design when the campaign revealed it in July, with one commenter asking how we would explain the suggestively interlocking T and P to our children.
Describing how we pick up on sarcasm is sometimes difficult because it depends on a lot of shared knowledge. For example, a picture of a snowy scene with the caption "beautiful weather" might be read literally—unless one knows enough about the tweeter or Instagramer to understand that they prefer tropical beach vacations.
To tackle the problem of converting this kind of subtlety into something digital, the team turned to humans. Schifanella worked with researchersPaloma de Juan, Joel Tetreault and Liangliang Cao from Yahoo! (which funded most of the study), to create a crowdsourcing tool asking people from several English-speaking countries to tag social media posts as sarcastic or not. First they assessed text-only statements, then statements accompanied by images. The participants did not always agree as to which post was sarcastic but the researchers found that in most cases the presence of a visual image helped identify a backhanded message. And regardless of whether there was an image, linguistic cues that gave away sarcasm to the participants included wordplay—using "I looooove the weather" rather than "I love the weather"—and punctuation, exclamation points (!) in particular.
The researchers then wrote a computer algorithm that mathematically represented what the humans had taught them. This allowed a machine to use that baseline data to look at new posts and decide whether they were sarcastic. Using a combination of features, the machine picked up on the sarcasm 80 to 89 percent of the time. There was some variation in the results, depending on the platform—Twitter, Instagram or Tumblr—and in the type of features used to detect the sarcasm. For example, using only the visual semantics (mathematical representations of the way humans categorize images from large databases) the accuracy dropped to 61 percent.
Sign up for the Live Science daily newsletter now
Get the world’s most fascinating discoveries delivered straight to your inbox.
Improved computer-processing power and large social networks make this type of machine learning possible, according to Tetreault, who is now director of research at Grammarly, which offers an online grammar and spell-checking program. More powerful machines can better handle this kind of neural network–based learning, and social networks provide the data. Drawing an analogy with learning to play baseball, Tetreault says, "A kid watching a game [may] not know the rules, but eventually he watches it enough and he figures out that hitting the ball hard is good."
Other scientists in the field say the work is an important step toward helping computers understand natural language. "Irony or sarcasm requires a notion of context. It is quite different from spam or even [textual] sentiment analysis," says Byron Wallace, an assistant professor at Northeastern University's College of Computer and Information Science who was not involved in the Turin–Yahoo! project. "Trying to incorporate some notion of context; that's what's cool about this."
Computers acting more like humans—just what we needed.
This article was first published at ScientificAmerican.com. © ScientificAmerican.com. All rights reserved.
Follow Scientific American on Twitter @SciAm and @SciamBlogs. Visit ScientificAmerican.com for the latest in science, health and technology news.