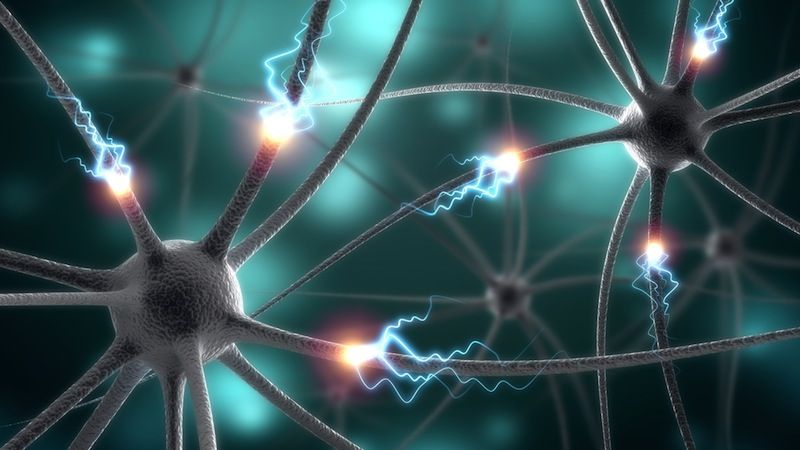
Spookily powerful artificial intelligence (AI) systems may work so well because their structure exploits the fundamental laws of the universe, new research suggests.
The new findings may help answer a longstanding mystery about a class of artificial intelligence that employ a strategy called deep learning. These deep learning or deep neural network programs, as they're called, are algorithms that have many layers in which lower-level calculations feed into higher ones. Deep neural networks often perform astonishingly well at solving problems as complex as beating the world's best player of the strategy board game Go or classifying cat photos, yet know one fully understood why.
It turns out, one reason may be that they are tapping into the very special properties of the physical world, said Max Tegmark, a physicist at the Massachusetts Institute of Technology (MIT) and a co-author of the new research.
The laws of physics only present this "very special class of problems" — the problems that AI shines at solving, Tegmark told Live Science. "This tiny fraction of the problems that physics makes us care about and the tiny fraction of problems that neural networks can solve are more or less the same," he said. [Super-Intelligent Machines: 7 Robotic Futures]
Deep learning
Last year, AI accomplished a task many people thought impossible: DeepMind, Google's deep learning AI system, defeated the world's best Go player after trouncing the European Go champion. The feat stunned the world because the number of potential Go moves exceeds the number of atoms in the universe, and past Go-playing robots performed only as well as a mediocre human player.
But even more astonishing than DeepMind's utter rout of its opponents was how it accomplished the task.
"The big mystery behind neural networks is why they work so well," said study co-author Henry Lin, a physicist at Harvard University. "Almost every problem we throw at them, they crack."
Sign up for the Live Science daily newsletter now
Get the world’s most fascinating discoveries delivered straight to your inbox.
For instance, DeepMind was not explicitly taught Go strategy and was not trained to recognize classic sequences of moves. Instead, it simply "watched" millions of games, and then played many, many more against itself and other players.
Like newborn babies, these deep-learning algorithms start out "clueless," yet typically outperform other AI algorithms that are given some of the rules of the game in advance, Tegmark said.
Another long-held mystery is why these deep networks are so much better than so-called shallow ones, which contain as little as one layer, Tegmark said. Deep networks have a hierarchy and look a bit like connections between neurons in the brain, with lower-level data from many neurons feeding into another "higher" group of neurons, repeated over many layers. In a similar way, deep layers of these neural networks make some calculations, and then feed those results to a higher layer of the program, and so on, he said.
Magical keys or magical locks?
To understand why this process works, Tegmark and Lin decided to flip the question on its head.
"Suppose somebody gave you a key. Every lock you try, it seems to open. One might assume that the key has some magic properties. But another possibility is that all the locks are magical. In the case of neural nets, I suspect it's a bit of both," Lin said.
One possibility could be that the "real world" problems have special properties because the real world is very special, Tegmark said.
Take one of the biggest neural-network mysteries: These networks often take what seem to be computationally hairy problems, like the Go game, and somehow find solutions using far fewer calculations than expected.
It turns out that the math employed by neural networks is simplified thanks to a few special properties of the universe. The first is that the equations that govern many laws of physics, from quantum mechanics to gravity to special relativity, are essentially simple math problems, Tegmark said. The equations involve variables raised to a low power (for instance, 4 or less). [The 11 Most Beautiful Equations]
What's more, objects in the universe are governed by locality, meaning they are limited by the speed of light. Practically speaking, that means neighboring objects in the universe are more likely to influence each other than things that are far from each other, Tegmark said.
Many things in the universe also obey what's called a normal or Gaussian distribution. This is the classic "bell curve" that governs everything from traits such as human height to the speed of gas molecules zooming around in the atmosphere.
Finally, symmetry is woven into the fabric of physics. Think of the veiny pattern on a leaf, or the two arms, eyes and ears of the average human. At the galactic scale, if one travels a light-year to the left or right, or waits a year, the laws of physics are the same, Tegmark said.
Tougher problems to crack
All of these special traits of the universe mean that the problems facing neural networks are actually special math problems that can be radically simplified.
"If you look at the class of data sets that we actually come across in nature, they're way simpler than the sort of worst-case scenario you might imagine," Tegmark said.
There are also problems that would be much tougher for neural networks to crack, including encryption schemes that secure information on the web; such schemes just look like random noise.
"If you feed that into a neural network, it's going to fail just as badly as I am; it's not going to find any patterns," Tegmark said.
While the subatomic laws of nature are simple, the equations describing a bumblebee flight are incredibly complicated, while those governing gas molecules remain simple, Lin added. It's not yet clear whether deep learning will perform just as well describing those complicated bumblebee flights as it will describing gas molecules, he said.
"The point is that some 'emergent' laws of physics, like those governing an ideal gas, remain quite simple, whereas some become quite complicated. So there is a lot of additional work that needs to be done if one is going to answer in detail why deep learning works so well." Lin said. "I think the paper raises a lot more questions than it answers!"
Original article on Live Science.
Tia is the managing editor and was previously a senior writer for Live Science. Her work has appeared in Scientific American, Wired.com and other outlets. She holds a master's degree in bioengineering from the University of Washington, a graduate certificate in science writing from UC Santa Cruz and a bachelor's degree in mechanical engineering from the University of Texas at Austin. Tia was part of a team at the Milwaukee Journal Sentinel that published the Empty Cradles series on preterm births, which won multiple awards, including the 2012 Casey Medal for Meritorious Journalism.