5 Questions for the Man Who Plans to Build a Brain
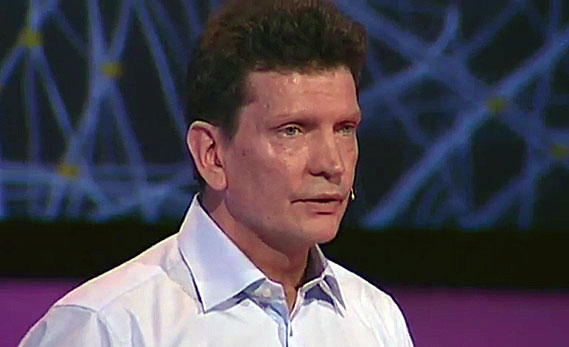
Henry Markram plans to build a virtual model of a human brain. A neuroscientist at the Swiss Federal Institute of Technology, he believes the only way to truly understand how our brains work — and why they often don't — is to create a replica out of 1s and 0s, then subject it to a barrage of computer-simulated experiments.
Markram has established the Human Brain Project to do just that. The effort aims to integrate all aspects of the human brain that have been discovered by neuroscientists over the past few decades, from the structures of ion channels to the mechanisms of conscious decision-making, into a single supercomputer model: a virtual brain. The project, which is controversial among neuroscientists, has been selected as a finalist for the European Union's two new Flagship Initiatives — grants worth 1 billion euros ($1.3 billion) apiece.
If Markram receives the funding, what exactly will he do, and why? We caught up with him to find out.
LLM: Do you already have a rough idea of how to build the brain, and if so, what’s the basic plan?
HM: Of course. We already have prototype systems in place, ready to expand, refine and perfect. There are a number of general principles and strategies that we apply. We start at microcircuits of neurons (a few tens of thousands of neurons) with morphological/geometrical detail and on this foundation we then move in two directions: We scale up toward the whole brain, and we increase the resolution of the neurons, synapses and in the future will add glial (non-neuronal cells) and blood flow models.
The models serve to integrate biological data systematically and therefore they can only get more and more accurate with time as they take more and more biological data into account — like a sponge. It is a systematic one-way track. We mine all existing data in the literature and in databases … organize the results, and analyze it for patterns and its value in helping to specify models more and more biologically accurately.
We develop [statistical] models that can be used to make predictions across gaps in our knowledge … and then use the simulations to test and improve these predictions. This strategy means that one will not have to measure everything in the brain to be able to build accurate models. When we identify gaps in knowledge that cannot be filled by prediction and that are crucial for building the models, we either do the experiments ourselves or we collaborate with or encourage someone to do the experiment. Sometimes we just have to wait for the data, but we keep building the software as if the data is there with place holders so we can integrate the data when it is obtained. [More on How to Build a Brain]
Sign up for the Live Science daily newsletter now
Get the world’s most fascinating discoveries delivered straight to your inbox.
LLM: When the brain is complete, will it actually think and behave like a human?
HM: Most likely not in the way you would imagine … When one builds a model like this it still has to be taught to sense, act and make decisions. That is a slow process and will need extremely powerful supercomputers. We will do that in a closed loop with virtual agents behaving in virtual worlds, but they will learn in slow motion, even on an exascale supercomputer (billion billion calculations per second) … We will also not have enough supercomputing power to simulate the brain at molecular level in every cell, but we aim to build multi-scale models and make supercomputers capable of simulating such multi-scale models that will allow more active neurons to run at higher resolution. Once we have this in place, it is mainly a matter of supercomputers getting more and more powerful and the models will automatically run at greater and greater levels of detail. No one knows what level of detail is needed in brain models to support cognitive tasks. Many hope and believe that it is enough for models to be simple models … We'll have to wait and find out.
For these reasons, early-version human brain models would be nowhere near as intelligent as humans. For some special tasks, maybe (like today's computers playing chess and "Jeopardy!"); this depends if we can work out the key computing principles behind specialized tasks. This will help us develop theoretical models that may be able to perform some specialized or focused tasks far better than humans. For example, they could make decisions on very large numbers of simultaneous input streams, like watching many movies at the same time. We would get completely lost and confused, but a computer brain model could potentially be trained to look for special relationships across all the movies.
LLM: How will the computer-brain relate to the outside world?
HM: We connect the brain models to virtual agents behaving in virtual worlds. Once the models can be simplified, then we will be able to build them into computer chips. These chips will be able to serve as a brain for physical robots and all kinds of devices. They will have to learn as the robot tries to do things. Such brain models will most likely not be anywhere near as powerful as the human brain, but they will probably be far more capable than any artificial intelligence system or robot that exists today. [Could a 'Robocopalypse' Wipe Out Humans?]
LLM: What’s the biggest challenge faced by the Human Brain Project, besides getting funding?
HM: The speed that we can run along our road map depends on how fast we can integrate the existing biological data, how many of the gaps we can fill in our knowledge using [statistical] predictions, how long it will take to get the data from key missing experiments that we cannot [statistically] leap over, the capability of the software that we build (it has to be able to capture biology with exquisite accuracy), the amount of computing power we can afford to buy, and the amount of computing power that will be available in the future. For computer science, the biggest challenge is to make supercomputers interactive just like a real-time scientific instrument.
LLM: What will the brain model be used for?
HM: It will be like a new instrument that can be used to look deep into the brain and across all the levels of biology (genes, molecules, cells, neuronal microcircuits, brain regions, brain systems to the whole brain — top to bottom, bottom to top) and see how all the components work together to allow our remarkable capabilities to emerge. It is the Hubble telescope for the brain. It will allow many scientists to work together on building the brain models, like the physicists do at CERN.
We don't have an X-ray multilevel view of the brain today and no amount of experiments will give us such a view anytime soon, so we do have to build this view if we want to understand the brain. We will use this multilevel view together with experimental data to begin to unravel the mysteries of the brain. We will be able to provide simulated data that can't be obtained experimentally and theorists will need to develop new theories of how the brain works.
There are around 560 brain diseases and we have very little hope of solving any of them with the current methods alone. With such a multilevel view of the brain we will be able to disrupt the brain model at any level (e.g. brain regions, connections, biological pathways, neurons, synapses, molecules and genes) and observe the effects. We will also be able to apply broken settings that have been worked out in experiments and study how the brain works differently to potentially cause the disease. In this way we will be able to search for the vulnerabilities of the brain and make a map of its weak points — all the serious places that could go wrong. So it will be a new instrument to help map out and study the brain's diseases. [Freakiest Medical Conditions]
Computing is hitting a wall with the traditional digital computing paradigm. It is hitting energy and robustness walls. Computers start to make more and more mistakes as they get faster and it is costing more and more energy to fix them. What will the new computing paradigm be? Quantum and other types of paradigms are probably several decades away. What is right here is what is called neuromorphic computing. The brain uses only around 20 watts, while the big computers of the future will need many megawatts. The brain is also extremely robust to mistakes and damage. For about 20 years now, the U.S., Europe and China have been developing the technology to build computer chips that can be configured with the network of a brain or a part of a brain. The problem is, no one has the networks. We only make a good guess at them today — a tough job when it took evolution billions of years to work out these intricate networks. In the Human Brain Project, we will be able to "export to neuromorphic"— export the network from the detailed models and configure these chips. The result could be a completely new generation of highly intelligent computers, electronic devices, and all kinds of information and communication systems — brainlike systems. This is a new paradigm for computing, for information and communication technologies.
Follow Natalie Wolchover on Twitter @nattyover. Follow Life's Little Mysteries on Twitter @llmysteries, then join us on Facebook.
Natalie Wolchover was a staff writer for Live Science from 2010 to 2012 and is currently a senior physics writer and editor for Quanta Magazine. She holds a bachelor's degree in physics from Tufts University and has studied physics at the University of California, Berkeley. Along with the staff of Quanta, Wolchover won the 2022 Pulitzer Prize for explanatory writing for her work on the building of the James Webb Space Telescope. Her work has also appeared in the The Best American Science and Nature Writing and The Best Writing on Mathematics, Nature, The New Yorker and Popular Science. She was the 2016 winner of the Evert Clark/Seth Payne Award, an annual prize for young science journalists, as well as the winner of the 2017 Science Communication Award for the American Institute of Physics.