To Track a Disease, Follow the Prom Kings and Queens
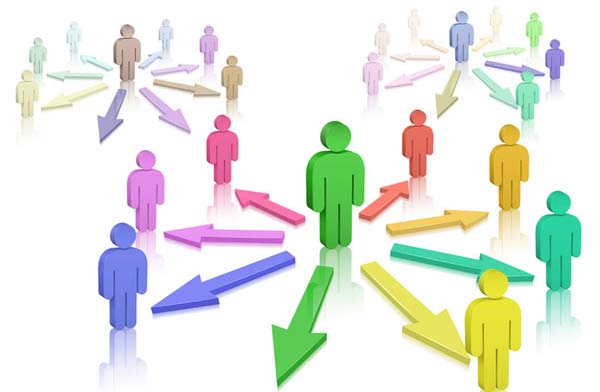
Scientists have come up with a new way to track and predict the spread of infectious diseases such as the flu: pinpoint the popular people.
Researchers know that, during a disease outbreak, the people at the center of a social network the prom kings, cheerleaders and class presidents of the world are likely to be infected sooner than the average Joe. These individuals have more contacts and are more likely to be the central figures in their social networks, so they come across gossip, ideas and, yes, germs more often than those at the outskirts of a social network.
By homing in on these popular people, scientists may find information about epidemics before the general population is stricken, according to study researchers Nicholas Christakis, a professor of medicine and sociology at Harvard University, and James Fowler, a professor of medical genetics and political science at the University of California, San Diego.
"If we could track those people, and figure out a way of monitoring the central people, then we would be able to get some kind of early warning about a disease that's spreading," Christakis said.
Friendship paradox
To do this, the researchers took advantage of a quirk of social networks known as the "friendship paradox." This paradox, Christakis says, posits that "your friends have more friends than you do." In other words, if you ask anyone to tell you the name of a friend, the nominated friend is likely to be more popular than the nominator is.
Christakis likened this paradox to a cocktail party to which a host invites 100 friends. If you ask people at the party to name a friend, many more guests will mention the host than mention a less-connected individual with only a few friends.
Sign up for the Live Science daily newsletter now
Get the world’s most fascinating discoveries delivered straight to your inbox.
To see if the friendship paradox could have been used to give early warning of the 2009 H1N1 flu epidemic at Harvard, the researchers threw such a "cocktail party."
They asked 396 Harvard students to name three friends each. After accounting for names that were nominated by more than one person, and discounting those nominees who didn't want to participate in the study, they ended up with a "friend group" of 425 people.
The researchers monitored both the original group and the friend group for symptoms and diagnosis of the flu throughout the last four months of 2009, relying on records from the University Health Services and self-reports of flu symptoms.
On average, the people in the friend group were infected by flu about two weeks before the people in the original group, according to the study. And when the researchers used computer models to try to forecast the epidemic that later struck by examining the data available on each day, they found they could detect the epidemic in the friend group 46 days before it peaked in the original group.
"By monitoring the friends of randomly chosen people, we were able to get not just rapid warning, but early detection of the epidemic," Christakis told MyHealthNewsDaily. "We could tell before the epidemic hit the general population, we knew it was coming."
Predicting an outbreak
Such a method of early detection would improve upon current methods for predicting disease spread, Christakis said. When public health officials gather reports of flu cases from the field, they can get a picture of the epidemic that's about two weeks delayed.
Other proposed ideas for monitoring the spread of disease, such as using Google Trends to track online searches about the flu, would let researchers know only where an epidemic is currently occurring; they could not predict the epidemic, Christakis said. But the friendship paradox could provide advanced detection of an epidemic, before it hits the general population, he said.
"It's a creative approach to a longstanding problem of how to improve disease surveillance," said Dr. Philip Polgreen, an assistant professor of medicine and epidemiology at the University of Iowa, who was not involved with the research.
"Surveillance data, almost by definition, it's old by the time it's available because there's some lag time. So approaches that help us even anticipate activity a week or two in advance are helpful," Polgreen said. This is particularly important since treatments for influenza are time-dependent, he said.
A next step might be to see if this approach works in other social networks made up of closed populations, such as military recruits, he said.
The friendship paradox could, in theory, be used to predict "outbreaks" of things including behaviors, norms and ideas. For instance, it might be able to predict an outbreak of self-injurious behavior among teenagers, Christakis said, a behavior that some evidence suggests is spread among peers.
The study was published today (Sept. 15) in the journal PLoS ONE.
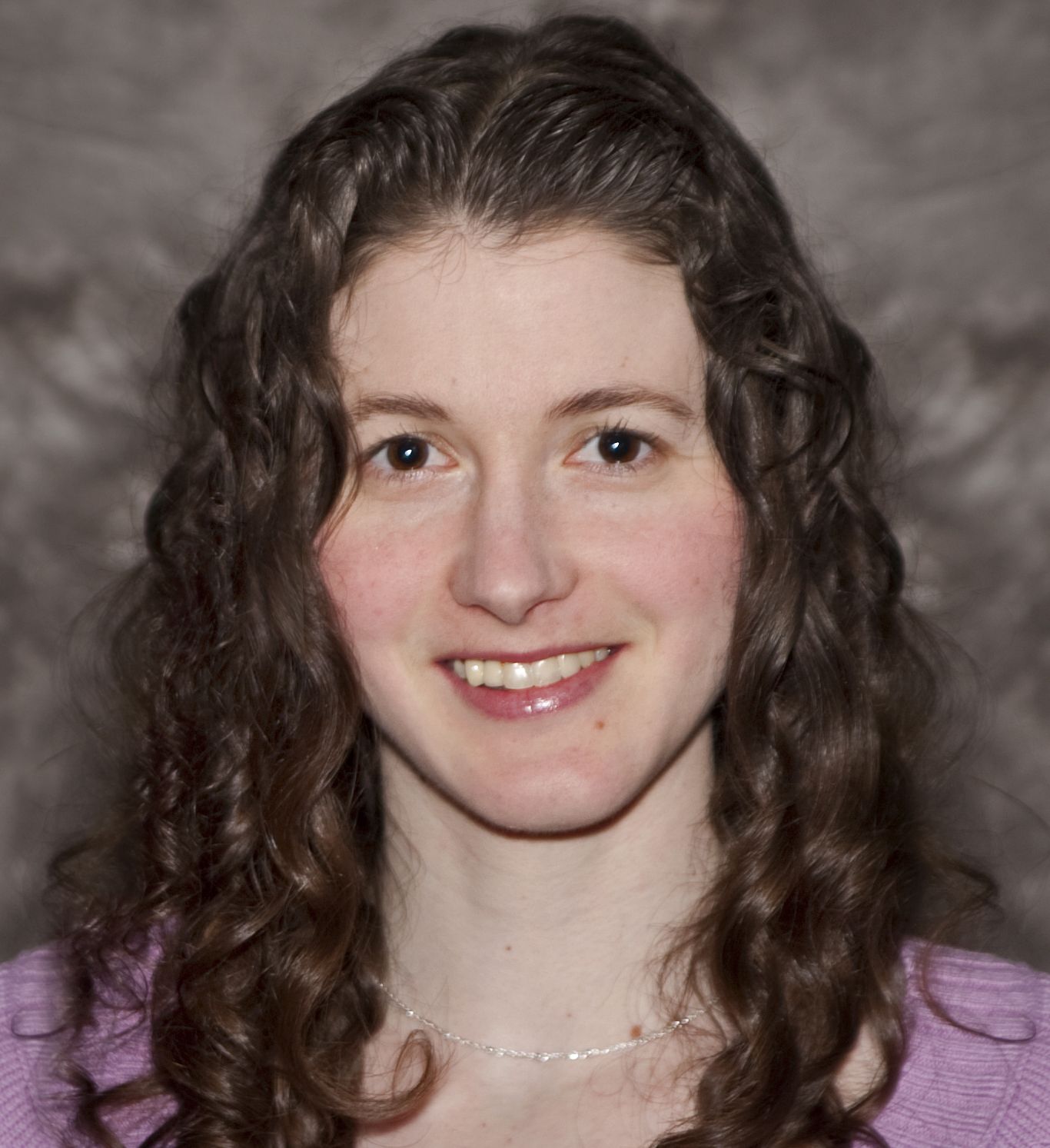
Rachael is a Live Science contributor, and was a former channel editor and senior writer for Live Science between 2010 and 2022. She has a master's degree in journalism from New York University's Science, Health and Environmental Reporting Program. She also holds a B.S. in molecular biology and an M.S. in biology from the University of California, San Diego. Her work has appeared in Scienceline, The Washington Post and Scientific American.