Forecasting Infectious Disease Spread with Web Data
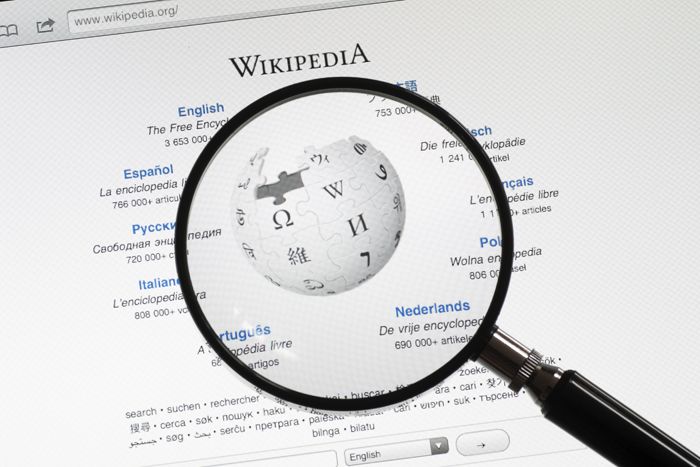
Just as you might turn to Twitter or Facebook for a pulse on what's happening around you, researchers involved in an infectious disease computational modeling project are turning to anonymized social media and other publicly available Web data to improve their ability to forecast emerging outbreaks and develop tools that can help health officials as they respond.
Mining Wikipedia Data
"When it comes to infectious disease forecasting, getting ahead of the curve is problematic because data from official public health sources is retrospective," says Irene Eckstrand of the National Institutes of Health, which funds the project, called Models of Infectious Disease Agent Study (MIDAS). "Incorporating real-time, anonymized data from social media and other Web sources into disease modeling tools may be helpful, but it also presents challenges."
To help evaluate the Web's potential for improving infectious disease forecasting efforts, MIDAS researcher Sara Del Valle of Los Alamos National Laboratory conducted proof-of-concept experiments involving data that Wikipedia releases hourly to any interested party. Del Valle's research group built models based on the page view histories of disease-related Wikipedia pages in seven languages. The scientists tested the new models against their other models, which rely on official health data reported from countries using those languages. By comparing the outcomes of the different modeling approaches, the Los Alamos team concluded that the Wikipedia-based modeling results for flu and dengue fever performed better than those for other diseases.
"We were able to use Wikipedia to forecast the number of people who may become sick in up to 4 weeks," explains Del Valle, who recently published results from a similar study that confirmed the potential of this approach to forecast seasonal flu spread.
Del Valle notes that the Wikipedia forecasting approach does have some limitations. For example, low Internet use in countries where certain diseases are endemic may help explain why her group's models of cholera performed less well than the ones of flu and dengue.
Developing the Apps
Sign up for the Live Science daily newsletter now
Get the world’s most fascinating discoveries delivered straight to your inbox.
"Studying how social media and related information can be appropriately and effectively used for infectious disease forecasting is also important," says Eckstrand.
Toward this end, the MIDAS group led by Stephen Eubank of Virginia Tech has been collaborating with a district epidemiologist in the Virginia Department of Health to test and potentially expand the application of a program called EpiDash.
EpiDash is a platform that uses machine-learning algorithms to screen anonymized public tweets for keywords related to flu, norovirus and even Lyme disease. Monitoring the rise and fall of tweets on a topic can aid efforts to identify and respond to emerging disease trends.
Like Del Valle, Eubank notes a variety of special considerations in using social media for disease monitoring and prediction efforts. These include technical hurdles, such as incorporating quickly changing hashtags or buzzwords, as well as privacy concerns. His group recently published an article that proposes ethical standards for research using Twitter data.
Digital data has helped MIDAS investigator Lauren Ancel Meyers of the University of Texas at Austin build an influenza monitoring system called the Texas Pandemic Flu Toolkit, a suite of online tools that Texas health officials can use to evaluate the potential effectiveness of different interventions such as antiviral drugs, vaccines and school closures.
The MIDAS researchers agree that integrating novel sources of information, such as publicly available Web data, into computational modeling tools could revolutionize disease monitoring and forecasting. As Meyers says, "We're just at the tip of the iceberg."
The research reported in this article was funded in part by NIH under grants U01GM097658, U01GM070694 and U01GM087719.
This Inside Life Science article was provided to LiveScience in cooperation with the National Institute of General Medical Sciences, part of the National Institutes of Health.