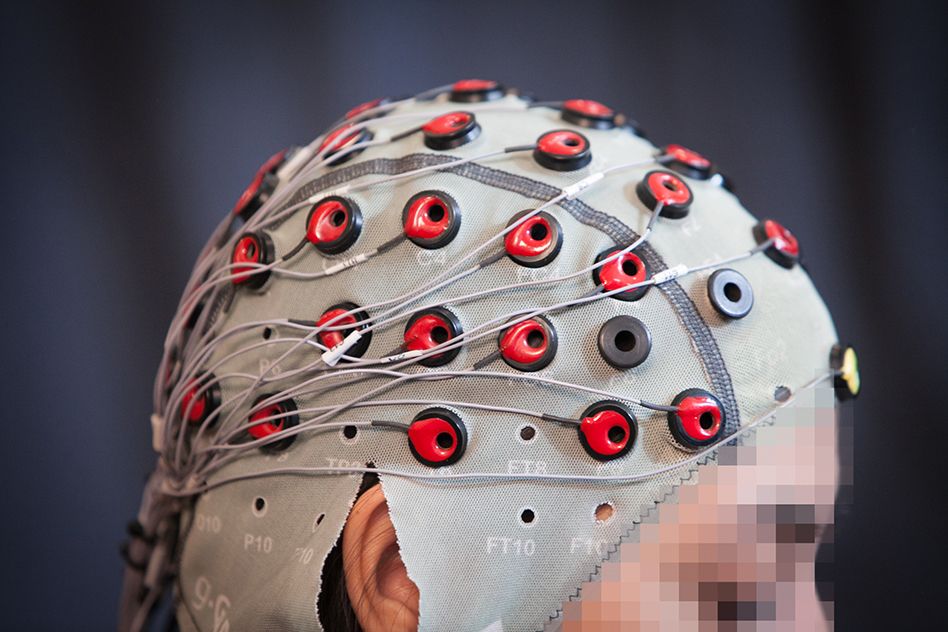
Are you nervous about entrusting your life to a self-driving car? What if you could telepathically communicate with the vehicle to instantaneously let it know if it makes a mistake?
That is the ultimate promise of technology being developed by a team fromBoston University and the Computer Science and Artificial Intelligence Laboratory (CSAIL) at the Massachusetts Institute of Technology. The tech uses brain signals to automatically correct a robot's errors.
Using a so-called brain-computer interface (BCI) to communicate with a robot is not new, but most methods require people to train with the BCI and even learn to modulate their thoughts to help the machine understand, the researchers said. [The 6 Strangest Robots Ever Created]
By relying on brain signals called "error-related potentials" (ErrPs) that occur automatically when humans make a mistake or spot someone else making one, the researchers' approach allows even complete novices to control a robot with their minds, the researchers in the new study said. This can be done by simply agreeing or disagreeing with whatever actions the bot takes, the researchers said.
Working with machines
This technology could offer an intuitive and instantaneous way of communicating with machines, for applications as diverse as supervising factory robots to controlling robotic prostheses, the researchers said.
"When humans and robots work together, you basically have to learn the language of the robot, learn a new way to communicate with it, adapt to its interface," said Joseph DelPreto, a Ph.D. candidate at CSAIL who worked on the project.
"In this work, we were interested in seeing how you can have the robot adapt to us rather than the other way around," he told Live Science.
Sign up for the Live Science daily newsletter now
Get the world’s most fascinating discoveries delivered straight to your inbox.
The new research was published online Monday (March 6) and will be presented at the IEEE International Conference on Robotics and Automation (ICRA) in Singapore this May. In the study, the researchers described how they collected electroencephalography (EEG) data from volunteers as those individuals watched a common type of industrial humanoid robot, called Baxter, decide which of two objects to pick up.
This data was analyzed using machine-learning algorithms that can detect ErrPs in just 10 to 30 milliseconds. This means results could be fed back to the robot in real time, allowing it to correct its course midway, the researchers said.
Refining the system
The system's accuracy needs significant improvement, the team admitted. In real-time experiments, the bot performed only slightly better than 50/50, or chance, when classifying brain signals as ErrPs. That meant that nearly half the time it would fail to notice the correction from the observer.
And even in more leisurely, offline analysis, the system still got it right only roughly 65 percent of the time, the researchers said.
But when the machine missed an ErrP signal and failed to correct its course (or change course when there was no ErrP), the human observer typically produced a second, stronger ErrP, said CSAIL research scientist Stephanie Gil.
"When we analyze that offline, we found that the performance boosts by a lot, as high as 86 percent, and we estimate we could get this upwards of 90 percent in the future. So our next step is to actually detect those in real time as well and start moving closer towards our goal of actually controlling these robots accurately and reliably on the fly," Gil told Live Science. [Bionic Humans: Top 10 Technologies]
Doing this will be tricky, though, because the system needs to be told when to look out for the ErrP signal, the researchers said. At present, this is done using a mechanical switch that gets activated when the robot's arm starts to move.
A secondary error won’t be created until after the robot's arm is already moving, so this switch won't be able to signal to the system to look for an ErrP, the researchers said. This means the system will have to be redesigned to provide another prompt, they added.
Now what?
The study is well-written, said Klaus-Robert Müller, a professor at the Technical University of Berlin, who was not involved with the new research but has also worked on BCIs that exploit these error signals. But, he said using ErrPs to control machines is not particularly new and he also raises concerns about the low ErrP classification ratesthe group achieved.
José del R. Millán, an associate professor at the École Polytechnique Fédérale de Lausanne in Switzerland, said he agrees that the performance of the group's ErrP decoder was low. But he thinks the approach they've taken is still "very promising," he added.
Millán's group has used ErrP signals to teach a robotic arm the best way to move to a target location. In a 2015 study published in the journal Scientific Reports, Millán and his colleagues described how the arm in their work starts by making a random movement, which the human observer decides is either correct or incorrect.
Through a machine-learning approach called reinforcement learning, the error signals are used to fine-tune the robot's approach, enabling the bot to learn the best movement strategy for a specific target. Millán said using ErrP to control robots could have broad applications in the future.
"I see it in use for any complex human-machine interaction where most of the burden is on the machine side, because of its capacity to do tasks almost autonomously, and humans are simply supervising," he said.
Original article on Live Science.