AI 'brain decoder' can read a person's thoughts with just a quick brain scan and almost no training
An improvement to an existing AI-based brain decoder can translate a person's thoughts into text without hours of training.
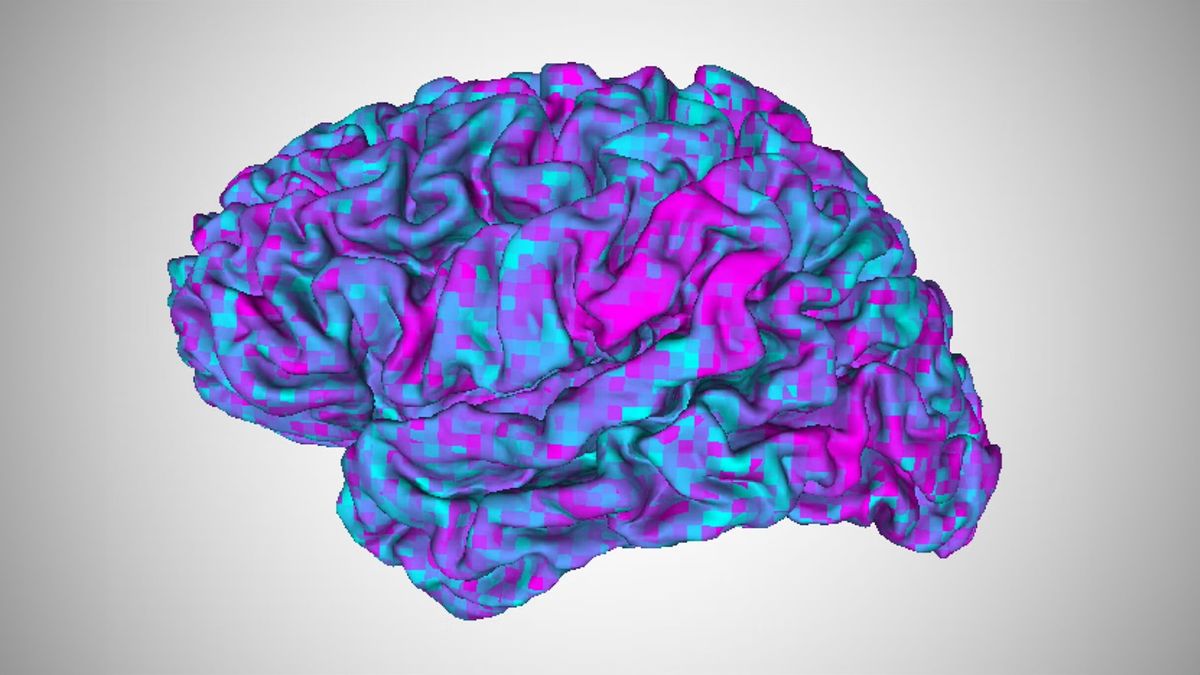
Scientists have made new improvements to a "brain decoder" that uses artificial intelligence (AI) to convert thoughts into text.
Their new converter algorithm can quickly train an existing decoder on another person's brain, the team reported in a new study. The findings could one day support people with aphasia, a brain disorder that affects a person's ability to communicate, the scientists said.
A brain decoder uses machine learning to translate a person's thoughts into text, based on their brain's responses to stories they've listened to. However, past iterations of the decoder required participants to listen to stories inside an MRI machine for many hours, and these decoders worked only for the individuals they were trained on.
"People with aphasia oftentimes have some trouble understanding language as well as producing language," said study co-author Alexander Huth, a computational neuroscientist at the University of Texas at Austin (UT Austin). "So if that's the case, then we might not be able to build models for their brain at all by watching how their brain responds to stories they listen to."
In the new research, published Feb. 6 in the journal Current Biology, Huth and co-author Jerry Tang, a graduate student at UT Austin investigated how they might overcome this limitation. "In this study, we were asking, can we do things differently?" he said. "Can we essentially transfer a decoder that we built for one person's brain to another person's brain?"
The researchers first trained the brain decoder on a few reference participants the long way — by collecting functional MRI data while the participants listened to 10 hours of radio stories.
Then, they trained two converter algorithms on the reference participants and on a different set of "goal" participants: one using data collected while the participants spent 70 minutes listening to radio stories, and the other while they spent 70 minutes watching silent Pixar short films unrelated to the radio stories.
Sign up for the Live Science daily newsletter now
Get the world’s most fascinating discoveries delivered straight to your inbox.
Using a technique called functional alignment, the team mapped out how the reference and goal participants' brains responded to the same audio or film stories. They used that information to train the decoder to work with the goal participants' brains, without needing to collect multiple hours of training data.
Next, the team tested the decoders using a short story that none of the participants had heard before. Although the decoder's predictions were slightly more accurate for the original reference participants than for the ones who used the converters, the words it predicted from each participant's brain scans were still semantically related to those used in the test story.
For example, a section of the test story included someone discussing a job they didn’t enjoy, saying “I’m a waitress at an ice cream parlor. So, um, that’s not…I don’t know where I want to be but I know it’s not that.” The decoder using the converter algorithm trained on film data predicted: “I was at a job I thought was boring. I had to take orders and I did not like them so I worked on them every day.” Not an exact match — the decoder doesn’t read out the exact sounds people heard, Huth said — but the ideas are related.
"The really surprising and cool thing was that we can do this even not using language data," Huth told Live Science. "So we can have data that we collect just while somebody's watching silent videos, and then we can use that to build this language decoder for their brain."
Using the video-based converters to transfer existing decoders to people with aphasia may help them express their thoughts, the researchers said. It also reveals some overlap between the ways humans represent ideas from language and from visual narratives in the brain.
"This study suggests that there's some semantic representation which does not care from which modality it comes," Yukiyasu Kamitani, a computational neuroscientist at Kyoto University who was not involved in the study, told Live Science. In other words, it helps reveal how the brain represents certain concepts in the same way, even when they’re presented in different formats.,
The team's next steps are to test the converter on participants with aphasia and "build an interface that would help them generate language that they want to generate," Huth said.
Skyler Ware is a freelance science journalist covering chemistry, biology, paleontology and Earth science. She was a 2023 AAAS Mass Media Science and Engineering Fellow at Science News. Her work has also appeared in Science News Explores, ZME Science and Chembites, among others. Skyler has a Ph.D. in chemistry from Caltech.
You must confirm your public display name before commenting
Please logout and then login again, you will then be prompted to enter your display name.