Tiny AI chip modeled on the human brain set to boost battery life in smart devices
The Spiking Neural Processor T1 is an AI chip that's modeled on the way the brain detects patterns and could extend the battery life in smart devices.
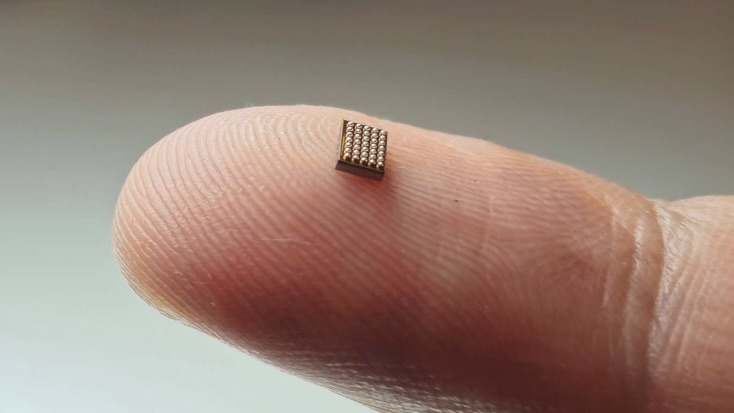
LAS VEGAS — The world's first "neuromorphic chip" will be on shelves by next year — and it will extend smart devices' battery life. The chip, which mimics the human brain's architecture, is meant to enable artificial intelligence (AI) capabilities on power-limited smart devices.
"Smart" devices like lightbulbs, doorbells or smoke alarms that are Wi-Fi connected are built with sensors that make detections and send data to the cloud for processing.
But this process is power-hungry, Sumeet Kumar, CEO of processor company Innatera Nanosystems, told Live Science in an interview at CES 2025. And any AI processing these devices perform also requires an internet connection.
But the Spiking Neural Processor T1 should drastically slash the power consumption of future smart devices.
It works by analyzing sensor data in real time to identify patterns and potentially clean up the data coming out of the sensors — and no internet connection would be required.
Mimicking the brain
The device is a neuromorphic processor — meaning its architecture is arranged to mimic the brain's pattern-recognition mechanisms. To draw an analogy, when you sense something — whether it's a smell or a sound — different collections of neurons fire to identify it.
Similarly, in the chip, different groups of artificial neurons register spikes. The underlying principle is the spiking neural network (SNN) — where a neural network is a collection of machine learning algorithms and the spikes it produces are akin to the signals produced by brain cells.
Sign up for the Live Science daily newsletter now
Get the world’s most fascinating discoveries delivered straight to your inbox.
Related: Intel unveils largest-ever AI 'neuromorphic computer' that mimics the human brain
SNN algorithms also tend to be around 100 times smaller in terms of file size than conventional deep neural networks used in large language models.
Layers of computation
There are three fundamental layers in the T1 chip. The first is the SNN-based computing engine, which records a power dissipation of less than 1 milliwatt and latency, or delay, that's typically under 1 millisecond for most applications, Kumar said. The second layer includes conventional deep neural networks, while the third layer includes a standard processor that handles how the system functions.
The T1, or similar chips, would increase battery life up to sixfold in some smart devices and scenarios, Kumar said. For example, a prototype of a smart doorbell built with the T1 processor that could detect the presence of a person using radar technology lasted 18 to 20 hours, versus one or two hours in a conventional Wi-Fi-based product that sends image and video data to servers.
Applications include smart lighting, any kind of detectors for people-counting, door-opening systems, and even earbuds — in which the T1 chip can theoretically isolate different sounds for noise cancellation. When used for any sound-based applications, the company claims there is an 80 to 100 times reduction in energy consumption as well as a 70 times reduction in latency.
The chip is being readied for mass production this year, with samples shipping to device manufacturers. Kumar expects the first products with the T1 neuromorphic chip to hit the shelves by 2026.
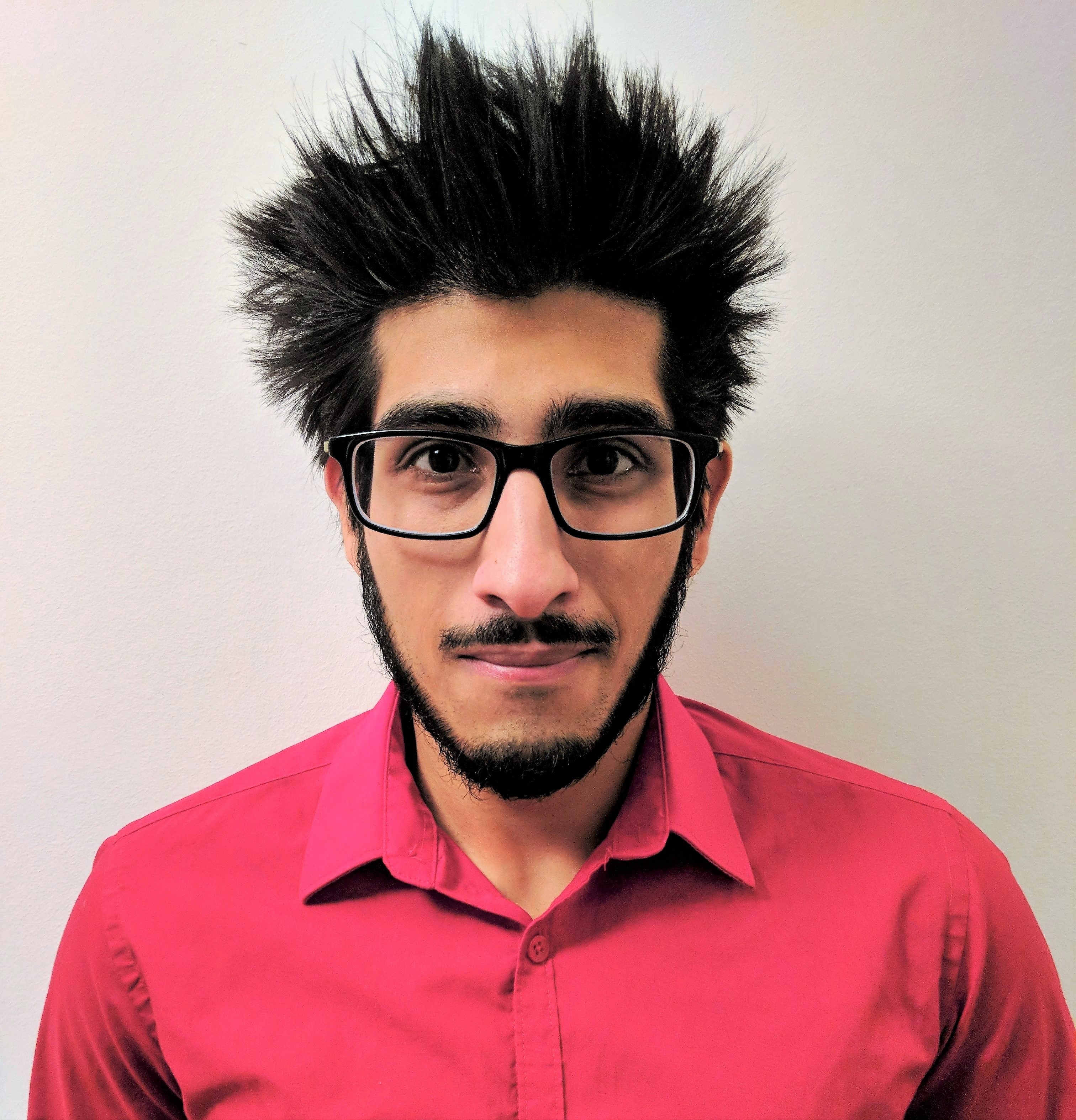
Keumars is the technology editor at Live Science. He has written for a variety of publications including ITPro, The Week Digital, ComputerActive, The Independent, The Observer, Metro and TechRadar Pro. He has worked as a technology journalist for more than five years, having previously held the role of features editor with ITPro. He is an NCTJ-qualified journalist and has a degree in biomedical sciences from Queen Mary, University of London. He's also registered as a foundational chartered manager with the Chartered Management Institute (CMI), having qualified as a Level 3 Team leader with distinction in 2023.