What is DeepMind?
DeepMind is an artificial intelligence technology that uses neural networks and machine learning to crack a broad range of problems, from protein folding to the game Go.
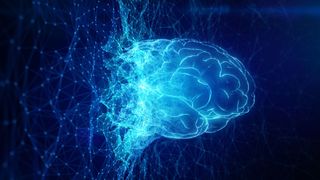
DeepMind is an artificial intelligence technology that uses machine learning to solve problems that computers haven't traditionally been able to tackle, such as beating humans at the game Go and predicting the myriad ways in which proteins can fold themselves into functional shapes. DeepMind's tech is already used in real-world applications. For example, it plays a role in slashing energy use at computing data centers and optimizing phone battery life.
The company DeepMind began as a London-based startup in 2010 and was acquired by Google in 2014. It's now a subsidiary of Alphabet Inc., the parent company of Google.
In September 2022, scientists from DeepMind won the $3 million Breakthrough Prize for their work on the protein-prediction program AlphaFold.
How does DeepMind work?
DeepMind's system is an artificial neural network. That means it's organized as a network of nodes, mimicking the way neurons connect to one another in the brain. Specifically, DeepMind uses a convolutional neural network, which is organized similarly to the human visual cortex, the part of the brain that processes visual information. The advantage of this kind of network is that, using a series of filters and large amounts of training data, the system can pick out particular features from those data. For instance, in image recognition, certain nodes become adept at recognizing a specific feature — for example, an eye or, in audio data, a particular combination of sounds.
Deep neural networks like DeepMind do this by running data through a series of layers known as "hidden layers." Each layer assigns weights to the data, essentially picking and choosing what the network will focus on, according to IBM. DeepMind has several hidden layers.
The first, the convolutional layer, detects features of the input using a filter known as a "kernal." The combination of the input and the kernel magnifies features that the algorithm deduces are important.
Related: Inductive vs. deductive reasoning
The next layer, known as the pooling layer, essentially reduces the complexity of the feature maps created by the convolutional layer, making it easier to process the data. Finally, the fully connected layer uses the output of the pooling layer to make future predictions. If, for example, the convolutional neural network has learned to recognize tumors in a set of medical training images, it can now take in new images and determine whether any tumors are present.
Because DeepMind is a machine-learning algorithm, it doesn't have to be given specific rules, written by programmers, to "learn." Instead, the algorithm is capable of combing through massive amounts of data and identifying repeated patterns that would take a human or traditional computer enormous amounts of time to decipher.
What does DeepMind do?
The advantage of DeepMind's machine-learning algorithms is that they can be used for all sorts of processes. DeepMind's algorithms can teach themselves to play Atari games and beat humans in Go, a stunningly complex strategy game that involves capturing territory on a gridded board using the placement of black-and-white pieces.
These games demonstrate the depth of the AI's ability to learn. DeepMind has since moved to tackling more and more real-world problems. These range from generating new pure-mathematics conjectures, which could speed the progress of theoretical math, to unraveling the likely structures of every protein known from nature.
The protein work, completed by the Breakthrough Prize-winning program AlphaFold, represented a massive change in the field of proteomics, the study of proteins. Proteins twist themselves into an array of shapes, but predicting how these internal structures will form is slow, painstaking work. Until AlphaFold, scientists had to freeze and image proteins using a method called X-ray crystallography. Decades of work had yielded structures for about 190,000 proteins. Within about a year, AlphaFold made predictions for 200 million protein structures.
DeepMind's technology is used at Google's data centers, where it controls the cooling of equipment while minimizing energy usage, according to the company. The DeepMind product WaveNet controls the voice of Google Assistant, and the company's AI is embedded throughout YouTube, controlling ad placement and other aspects of the video platform.
In 2022, researchers at the Swiss Federal Institute of Technology in Lausanne (EPFL) reported that, in collaboration with DeepMind, they'd tested the company's AI to shape hydrogen plasma inside a fusion reactor — a step toward using nuclear fusion as an energy source. Company researchers are also working on applying algorithms to self-driving cars, sports analysis and medical diagnoses.
What records has DeepMind broken?
AlphaFold's record-breaking speed at predicting protein shapes isn't the only superlative DeepMind has achieved. In October 2022, the company broke a 50-year-old mathematics record. The record involved finding a new way to do matrix multiplication, or multiplying arrays of numbers with one another. Multiplying a 4-by-4 matrix of numbers with another 4-by-4 matrix takes 64 calculations written out by hand. In 1969, mathematician Volker Strassen developed an algorithm that could do it in 49 calculations. A DeepMind AI called DeepTensor, on the other hand, showed that it could do the job in just 47 calculations.
Shortly thereafter, another group of researchers posted a preprint paper revealing that they could also use DeepTensor to slice the number of calculations needed to multiply two 5-by-5 matrices together, from 96 to 95.
DeepMind has also repeatedly beat the world's leading players of Go, even spurring the retirement of South Korean champion Lee Se-dol in 2019. "With the debut of AI in Go games, I've realized that I'm not at the top even if I become the number one through frantic efforts," Lee told Korea's Yonhap news agency that year. DeepMind's AlphaGo beat Lee in four out of five games in a 2016 tournament, which actually makes Lee the only human ever to beat AlphaGo in competition. In 2017, AlphaGo again beat the reigning human champion of the game, China's Ke Jie.
Is DeepMind ethical?
Given its immense power to drive the social media algorithms that determine what information people see, to diagnose medical conditions that may be a matter of life or death, and perhaps one day to drive people's cars for them, DeepMind's creators bear a heavy responsibility.
DeepMind launched an Ethics & Society team in 2017, but this hasn't kept the company entirely out of trouble. DeepMind is currently facing a class-action lawsuit in England over its clinical safety testing of an app called Streams, which is designed to detect acute kidney injury. According to HealthcareITNews, the Royal Free London NHS Foundation Trust provided patient data for the testing, but it was later determined that the Trust broke the U.K.'s patient data protection law in doing so.
DeepMind's power to not just identify images, video and audio but also to create new, ultrarealistic versions of all three means that the AI could be used to worsen the disinformation problem that already plagues the internet. Critics have warned that DeepMind's AI can be used to create "deepfakes," which are extremely realistic CGI videos that seem to show real events. (DeepMind isn't the only AI that can do this; a collaboration between the Massachusetts Institute of Technology and two AI companies built a https://moondisaster.org/ giving the speech President Nixon would have given had the first crewed moon landing gone wrong.)
Regardless of whether DeepMind is ethical, the technology will certainly bring new issues to grapple with as it becomes more widespread.
Originally published on Live Science.
Sign up for the Live Science daily newsletter now
Get the world’s most fascinating discoveries delivered straight to your inbox.
Stephanie Pappas is a contributing writer for Live Science, covering topics ranging from geoscience to archaeology to the human brain and behavior. She was previously a senior writer for Live Science but is now a freelancer based in Denver, Colorado, and regularly contributes to Scientific American and The Monitor, the monthly magazine of the American Psychological Association. Stephanie received a bachelor's degree in psychology from the University of South Carolina and a graduate certificate in science communication from the University of California, Santa Cruz.